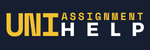
MBIS5013 Sustainability and Enterprise 4.0 Report 2 Sample
Assignment Details
In this assessment, you will work individually. You need to write a report on technologies that enable an organization to adopt a sustainable Enterprise 4.0 model. You can choose any number of technologies listed below to support your discussion:
1. Virtual Reality (VR) and Augmented Reality (AR) applications
2. Artificial Intelligence (AI)
3. Big Data and Analytics
4. Cloud-based Internet of Things (IoT)
5. 5G for Smart Cities
6. Blockchain
You are encouraged to attend a workshop on Referencing and Research Practice organised by the Academic Success Team (AST). You may also schedule a one-on-one workshop with the AST by emailing academicsuccess@aih.nsw.edu.au.
The final submission must include the following:
• A title page with information on the student, course, and assignment
• A table of contents
• A table of figures/graphs/illustrations etc (if required)
• An Executive Summary concisely explaining the aims, objectives and content of the report between 250 – 300 words
• The introduction to the overall report
• Main body of the report detailing concepts and ideas. This will contain material summarised from the articles you have read related to your topic with proper in-text references. The discussion in the main body can be organized in sections and subsections.
• A conclusion to the overall report
• A full reference list in APA style.
A single Microsoft Word document within 2500 words and written in size 12 Arial Font A minimum of eight scholarly journal articles must be used in your report You must submit your own work and use appropriate references in APA style where needed.
Solution
1.0 Introduction
Enterprise 4.0 has been identified to be beneficial for different IT companies, as it has introduced many of the advanced technologies. However, challenges have emerged due to this rapid introduction and adaptation by different organisations as well. Artificial intelligence is one of the most effective technologies that offers facilities such as predictive management, flawless production and planning, efficient logistics, inventory and many more. More importantly, smart manufacturing, which is possible to access through AI, has been a major component of enterprise 4.0. That is why this report aims to explore different aspects of the current IT market and evaluate the effectiveness of Infosys' AI implication and the results gathered.
2.0 Main Content
2.1 Concepts of sustainability and enterprise
Generally, sustainability for IT-based businesses and services has been identified as the combination of key aspects, such as social, environmental and economic (Toli & Murtagh, 2020, p.1). There can be differentiation in addressing the priorities of different individuals or the management. Therefore, it has been possible to understand that perceptions and practices, according to priorities, can be sustainable. On the other hand, there are certain limitations and barriers regarding technology-based services, which are also key components of the concept of sustainability. On the other hand, the most effective impact that artificial intelligence has brought to Enterprise 4.0 is digital financial inclusion (Mhlanga, 2020, p.1). This approach has facilitated sustainability, as even the people from the lower base of the social pyramid have become financially active. That is why the majority of IT-based companies have been seen to adopt AI to make their organisational activities sustainable, according to the demands of enterprise 4.0.
At the same time, in the case of Infosys, the AI location has been identified as quite suitable for providing integrated offerings. Therefore, it has been possible for Infosys to scale the demands of modern enterprises through futuristic AI-generated transformation (Infosys Limited, 2024). Furthermore, the implication of AI in Infosys has been identified as part of Infosys Topaz, which has been set to accomplish comprehensiveness. Different facilities, such as convergence of power, delivering modern business solutions, perceptive experiences and others, have been possible to attain through AI in Infosys. On the other hand, it has also been identified that AI-Cloud has been efficient for this organisation in terms of greater accessibility, deployment, and contextualization. These are the approaches that have enabled sustainability regarding the efficiency required for Enterprise 4.0.
2.2 Interconnection between natural environment, society and sustainability
Sustainability has been quite dependent on social development and the practices towards development. Therefore, it has been possible to understand the fact that complex thinking is crucial for social information that can be used to overcome a diversified range of limitations and gaps (Luna-Nemecio et al. 2020, p.1). Further understanding also clarifies the fact that social aspects play a crucial role in sustainability as well. Therefore, an interconnection can be seen between economic, socio-cultural and environmental mix to establish critical solutions. That is why, in this present time, technologies, such as artificial intelligence, have become quite popular for the capability of providing these types of solutions. Artificial intelligence is quite capable of measuring different variables at the same time while gathering a larger volume as well, which effectively considers systematic risks through detecting and adapting climate aspects (Galaz et al. 2021, p.1). The flawless, automated evaluation enables enhanced decision-making for a business or management to deliver aiding solutions for university assignment help.
As for Infosys, sustainability has been prioritised not just at the enterprise level but also in a broader social segment. Therefore, Infosys has adequately understood the importance of environmental, social and governance to include effective dimensions. Further effectiveness of this approach has been ensured through a combination of carbon mitigation and a service-based approach (Infosys, 2024, p.1). This specific approach of Infosys clearly defines the interconnection between the environment, society, and sustainability. As for better clarification, the approaches have been offered for an entire market, society, and communities, and the demands of Infosys clients have been fulfilled. Technological Implication in this process has allowed Infosys to segment into two timeframes, such as a 12-month goal and a 24-month goal. On the other hand, AI implication in Infosys has led the company to reduce energy consumption by up to 85% (Reddy et al. 2024, p.6). The cruciality of energy consumption regarding the after-effects on the environment, society, and other aspects has been identified through the critical thinking capability of AI.
2.3 Trends and challenges
Figure 1: Overall market size of AI
Source: (Statista, 2024)
Trends: Based on the current market trends and the adaptable intentions of different organisations, it has been estimated that the market size for AI will reach 184 billion dollars (Statista, 2024, p.1). At the same time, it also has been identified that the market will be able to continue with a growth rate of 28.46% from 2024 to 2030 (Statista, 2024, p.1). These estimations justify the trend of AI in the modern digitised market. There are different operational efficiencies that AI can offer, among which machine learning has been identified to be the most suitable one. That is why different IT companies are adopting machine learning to establish a suitable correlation between technological and human skill sets.
Challenges: Despite the multiple opportunities that artificial intelligence creates for a business, a lack of expertise in this technology can be seen to be effective for notable challenges (Kaggwa et al. 2024, p.424). Generally, AI is used within the IT business first for adequate capabilities for systematic and strategic decision-making and solution establishment. However, data biases within AI can be effective for IT firms in getting access to accurate solutions and decisions. On the other hand, it also has been seen that AI always requires a large amount of high-quality data sets, which is not possible for any IT company to provide all the time to guarantee accurate outcomes.
AI in Infosys: Generative AI has been seen to be one of the key trends that Infosys has continued following for closed-source models (Infosys, 2024, p.1). Furthermore, based on the applicability of generative AI, it has been possible for Infosys to establish a broader set of acceptance. As for greater implications, the foundational model has been identified as GPT-3.5/4/Turbo, PaLM, Anthropic and others (Infosys, 2024, p.1). These models within Infosys effectively boost operational activities while addressing the required implications, even for diverse segments of the business. At the same time, this trend regarding Generative AI in Infosys has been quite effective for different applicability’s, such as case management based on industry, augmented methods and others. The major aspect that can be highlighted for these situations is that the overall work can be completed even without fine-tuning, which has enabled a better trust towards AI.
2.4 Information System-based Theories
Information system research framework
Data exchange and automation are current trends in the IT industry that revolutionise the system with 4.0. Innovations. Information is transformed to flow from different sources, and this can be termed the theoretical foundation for ICT (Information Computer technology). Information flows from disparate data sources, resulting in new information. Interaction between the various systems grows complex, and this transfer process becomes critical, thus creating internal chaos across the systems (Shin, 2021). Different processes take action in regulated ways and produce definite results. The methods and tools are built to ensure that the systems continue to deliver comprehensive results.
Figure 2: Information system theory
Source: (Gregor, 2002)
This is the connection between people, organisations and technology. Infrastructure, communications, and applications help develop the appropriate environment. Technological agility is used across the proper climate to add to the knowledge base (Gregor, 2002, p.). Infosys explores various insights from the open-source database, exercises to curate data for building new models, and utilises the organisational case study. AI is also seen to be used in cybersecurity areas to safeguard the organisation from attackers. The activity of cyber attackers is eliminated, and threat detection is ensured more effectively.
TAM model
The technology acceptance model advocated in 1989 is also significant in predicting individual adoption. The application of the new technology grows on the perceived usefulness and ease of use. The application is easy to comprehend, and a high level of productivity affects social influences. The model helps measure the development of technological trends to show how experiences and effective technologies influence acceptance and are integrated to provide more reliable conditions.
Figure 3: TAM model
Source: (Miller & Khera, 2010, p.3)
This can be related to the AI utilisation on which Infosys's perceived usefulness is focused. AI clouds are negated across the value chains to apply their full potential. Infosys managers perform risks and manage reputational constraints to implement security and regulatory norms (Miller & Khera, 2010, p.3). Generative revolutions are led to offer the ethical, human and digital dimensions. Digital learning platforms also manage talent risks and pose a threat to traditional workplaces. The design frameworks are evolved to impact the data set along with the processes. Applied AI effectively empowers the system to bring in changes along the way.
2.5 Evaluate and reflect different perceptions to make changes
Automation acceleration: Infosys implements cutting-edge solutions to empower operational excellence and bring in aspiring growth. Regenerative AI offers immense benefits such as cost savings, resilience building and trust building. The clients develop trust in the automation process, which affects the implementation of data in human-centric ways. The application makes way for the effective use of resources and faster manufacturing. Innovation opportunities are created to give organisations a competitive edge (Shulner-Tal et al., 2024, p.2). AI monitors all the data in real time, and product quality is improved. The workforce gets safety and data-based tools to ensure quality in process and productivity. Human potential is amplified to unlock the value across organisations. Almost 80 % of workers think that AI makes them more productive. Platform engineering amplifies the development, and the software developers are offered the technologies to grow self-service platforms.
Revenue generation: The AI-centric model ensures that revenue generation and organisational effectiveness are grown at a scale. Product management is developed by optimising promotional decisions and merchandising. AI unlocks many of the untapped opportunities for revenues and enhances long-term values. Disruptive technologies are used to grow the initiatives, and business values are measured through the strategies (Khadpe et al., 2020. P.3). AI algorithms affect the indigenous solutions of AI to address various business problems. Clouds and analytics are leveraged to affect business solutions. There are the risks of digital disruptions that combat real-world challenges and arrive at decisions. Changes are fascinating in the system through resilient practices. The partner ecosystem is ever-growing, and the model of talent development is there that sets up the resilient process.
Responsible AI: Ethical AI captures the oversights throughout the development of the AI lifecycle. Continuous auditing processes are impacted through the active principles that ensure transparency and fairness. The adoption of AI advocates for legal and ethical concerns, and responsible AI helps development (Infosys, 2024, p.1). The approaches help generate unbiased and privacy-protected systems. AI implementation brings with it the challenges of navigating the regulatory norms. The regulation leads to disastrous outcomes in decision-making that are effective in adhering to the AI practices that develop AI solutions.
2.6 Recommendations for Infosys
? Infosys must seriously consider the self-governance framework. This is specifically for setting up the AI principles that give tangible solutions for creating responsible AI. The principles translate the ethics into actionable insights in making evaluations. The organising, again and again, cross-checks all the AI-general principles (Ragot et al., 2020, p.1). Output must be analysed to check against the organisational norms. The concepts must be dismissed as unsafe and the contextual assumptions must develop open models to question if the organisation is ready for stricter enforcement. Model adoption carries with it the indemnities and binding warranties. The organisations help in overcoming the challenges for the organisations. The evaluation is effective in rioting continuous development for generative AI and rigorous controls (Ragot et al., 2020, p.1). Data transparency must be there to ensure accountability for the consumers. Internet-specific regulations need to be efficient in regulating AI. The most effective approaches to AI are set up through the creation of AI-generated models.
? Make Advanced security to guarantee transparency in the AI design. The defences must be ensured to match the AI development speed. Trust must be present in the AI system generation to enable implementation at scales (Shin, 2020, p.102551). Susceptibility is a growing concern, and AI threats must be assessed. Attack on the deep learning models must be prevented through subtle approaches. The attacks are categorised as backdoor attacks, posing, inference, evasion and backdoor attacks. AI hygiene measures must be implemented to initiate the governing practices. Défense platforms are built to integrate the security system to affect the standardised operations. The guidelines become effective in witnessing the pervasive growth in securing AI benefits. Advanced AI must detect vulnerabilities and use security measures to ensure that systems do not fail. Predictive analysis becomes the core principle to get past poor security systems.
? Red teaming practices must be there to respond to effective behaviours. Organisational weaknesses and strengths must be detected to establish fairness, explainability and transparency. Network distillation is important in the model training phase (Shin, 2021, p.541). Su8sceptibility can be diminished, which can enhance the robustness of the model?. Data scientists must respond to anomalous behaviours to increase the organisation's strength and effectiveness. The organisations must be effective in shedding light on the detection of the strategies that reduce future threats.
3.0 Conclusion
Following the above discussion, Enterprise 4.0 has been effective in bringing an evolution in the IT industry. Rapid digitalisation has played a key part in this revolution, which has made different technologies more vital for companies. As for Infosys, artificial intelligence has been effective in offering different advantages. Based on current market trends and demands, Infosys has gained the capability to offer the most suitable solutions for sustainable developments. The social, environmental, and economic aspects have been possibly satisfied through implications such as carbon footprint mitigations. However, certain rooms for improvement can be obliged through self-governance, transparency and many more.
Reference List
Galaz, V., Centeno, M. A., Callahan, P. W., Causevic, A., Patterson, T., Brass, I., ... & Levy, K. (2021). Artificial intelligence, systemic risks, and sustainability. Technology in Society, 67, 101741. DOI: https://doi.org/10.1016/j.techsoc.2021.101741
Gregor, S. (2002). Design Theory in Information Systems. Australasian Journal of Information Systems, 10(1). https://doi.org/10.3127/ajis.v10i1.439
https://doi.org/10.1016/j.ijhcs.2020.102551
Infosys (2024) Solutions to enable climate change action among clients. https://www.infosys.com/about/esg/environmental/emission/solutions-enable.html#:~:text=We%20approach%20sustainability%20at%20the,reduction%20and%20science%2Dbased
%20offsetting.
Infosys Limited (2024) Infosys applied ai, Infosys. https://www.infosys.com/services/applied-ai.html
Infosys, I. (2024) Digital AI-first transformation and latest trends, Infosys. https://www.infosys.com/iki/techcompass/artificialintelligence.html
Infosys. (2024). Tech Navigator: Building the AI-First Organization. Infosys Germany - IT Business Services & Consulting - Overview. https://www.infosys.com/iki/research/tech-navigator.html
Kaggwa, S., Eleogu, T. F., Okonkwo, F., Farayola, O. A., Uwaoma, P. U., & Akinoso, A. (2024). AI in decision making: transforming business strategies. International Journal of Research and Scientific Innovation, 10(12), 423-444. DOI: https://doi.org/10.51244/IJRSI.2023.1012032
Khadpe, P., Krishna, R., Fei-Fei, L., Hancock, J. T., & Bernstein, M. S. (2020). Conceptual metaphors impact perceptions of human-AI collaboration. Proceedings of the ACM on Human-Computer Interaction, 4(CSCW2), 1-26. DOI: https://doi.org/10.1145/3415234
Luna-Nemecio, J., Tobón, S., & Juárez-Hernández, L. G. (2020). Sustainability-based on socioformation and complex thought or sustainable social development. Resources, Environment and Sustainability, 2, 100007. DOI: https://doi.org/10.1016/j.resenv.2020.100007
Mhlanga, D. (2020). Industry 4.0 in finance: the impact of artificial intelligence (ai) on digital financial inclusion. International Journal of Financial Studies, 8(3), 45. DOI: https://doi.org/10.3390/ijfs8030045
Miller, J., & Khera, O. (2010). Digital Library Adoption and the Technology Acceptance Model: A Cross-Country Analysis. The Electronic Journal of Information Systems in Developing Countries, 40(1), 1–19. DOI: https://doi.org/10.1002/j.1681-4835.2010.tb00288.x
Ragot, M., Martin, N., & Cojean, S. (2020, April). Ai-generated vs. human artworks. a perception bias towards artificial intelligence?. In Extended abstracts of the 2020 CHI conference on human factors in computing systems (pp. 1-10). DOI: 10.1145/3334480.3382892ff.
Reddy, M. S., Deepthi, S., Bhattaru, S., Srilakshmi, V., & Singh, H. (2024). Harmony in HR: Exploring the Synergy of Artificial Intelligence and Green Practices for Sustainable Workplaces”. In MATEC Web of Conferences (Vol. 392, p. 01039). EDP Sciences. DOI: https://doi.org/10.1051/matecconf/202439201039
Shin, D. (2020). User perceptions of algorithmic decisions in the personalized AI system: Perceptual evaluation of fairness, accountability, transparency, and explainability. Journal of Broadcasting & Electronic Media, 64(4), 541-565. DOI: https://doi.org/10.1080/08838151.2020.1843357
Shin, D. (2021). The effects of explainability and causability on perception, trust, and acceptance: Implications for explainable AI. International Journal of Human-Computer Studies, 146, 102551. DOI:
Shulner-Tal, A., Kuflik, T., Kliger, D., & Mancini, A. (2024). Who Made That Decision and Why? Users’ Perceptions of Human Versus AI Decision-Making and the Power of Explainable-AI. International Journal of Human–Computer Interaction, 1–18. https://doi.org/10.1080/10447318.2024.2348843
Statista (2024) Artificial Intelligence - Global: Statista market forecast, Statista. https://www.statista.com/outlook/tmo/artificial-intelligence/worldwide#:~:text=Artificial%20Intelligence%20%2D%20Worldwide&text=The%20market%20size%
20in%20the,US%24826.70bn%20by%202030.
Toli, A. M., & Murtagh, N. (2020). The concept of sustainability in smart city definitions. Frontiers in Built Environment, 6, 77. DOI: https://doi.org/10.3389/fbuil.2020.00077