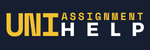
HI6037 Business Analytics Fundamentals Assignment Sample
Assignment Details
• All answers must be entered in the answer boxes provided after each question.
• Your assessment must be in MS Word format only.
• Reference sources must be cited in the text of the report and listed appropriately at the end in a reference list using Holmes Institute Adapted Harvard
Referencing. Penalties are associated with incorrect citation and referencing.
Question 1
1A: Describe the steps you would take to merge the cleaned_Location.xlsx and cleaned_Budget.csv datasets in Power BI. What key factors must you consider to ensure data integrity?
1B: Using the merged dataset, create a visualization in Power BI that displays the correlation between budget allocations and property price changes over time. Explain how your visualization can be interpreted.
Datasets Used:
1 cleaned_Location.xlsx
2 cleaned_Budget.csv
Question 2
2A: Explain how you would assess the accuracy of sales forecasts using the cleaned_Forecast.csv and cleaned_dim_tables_final.xlsx datasets. What specific Power BI functionalities would you use?
2B: Develop and describe a geographic visualization in Power BI that highlights sales distribution and high-performing regions. Include details on any filters or slicers you would add to enhance interactivity
Datasets Used:
cleaned_Forecast.csv
cleaned_dim_tables_final.xlsx
Question 3
3A: What variables have been included in this synthetic dataset for simulating tourism data? Can you briefly explain each one?
3B: Create a dynamic map in Power BI using your synthetic dataset that shows tourism density and its economic impact on local economies. Describe how you would set up this visualization to provide insights into peak times and spending patterns.
Datasets Used:
cleaned_Synthetic_Tourism_Data.xlsx
Question 4
4A: Discuss how you would analyze customer demographics, purchasing patterns, and profitability using the synthetic transaction data. What analytical techniques would you apply?
4B: Design a dashboard in Power BI to visualize distinct customer segments based on profitability and purchasing behavior. Explain how each element of the dashboard contributes to understanding customer segments.
Datasets Used:
cleaned_Synthetic_transaction_data.csv
Question 5
5A: Describe the process you would use in Power BI to cleanse and prepare a complex dataset for analysis. What challenges might you encounter and how would you address them?
5B: Prepare a presentation in Power BI to showcase findings from one of the datasets provided. Explain how you would use narrative elements and annotations to guide viewers through your analysis.
Datasets Used:
Any provided dataset (e.g., cleaned_Budget.csv)
Solution
Question 1A
To merge cleaned_Locationxlsx and cleaned_Budgetcsv in Power BI, first, it is required to import both tables: Pinpoint the position of the area/areas of concern in these fields and establish the main indexing instrument such as ‘LocationID.’ As subsequent merge is done based on this key, it is adequate to complete this process in the PQ Editor (Sharma et al. 2021, pp. 1-11). In making decisions here one is able to manage conflicts, freeze or expel members and make decisions regarding short comings concerning the quality of contents within the said set. It is also very crucial to highlight the fact that all the columns that you are to include in one should have the same data type. As for the other aspects concerning this process, they include; quality check of the data to be merged is conducted while missing values as well as synchronization of key fields is also done in order to have an efficient merge of data for university assignment help.
Question 1B
.png)
(Source: Power BI)
Looking at the budget on sum of comparison between 2020-2024, again the values showed negative up move indicating that the changes in property prices. So the actual reduction was 0.67% or 3425.40 units below 512,212.18 to 508786.78. This period was witnessed by the budgeting period which was lower than any other period indicating a lot of ignorance of the budget. The monthly percentage change in property prices was also lower, at 69% during the same period. Within the last few years, all the aspects other than the budget have set quite stable trends; the significant drop in the budget commenced in the year 2020. It has been seen that it has been on a decline every year for four consecutive years; it thus indicate changes that are major on budget priorities, which may not be independent but are most likely to be allied to other changes of an economic or an organisational nature.
Question 2A
To finally determine the degree of cleanliness of cleaned_Forecast, which denotes the extent of accuracy of the sales forecasts, the corresponding measure will be computed. csv and dim_tables. On Power BI, go through the Import section and joinery processes to enrich the forecast data for the analysis with the reference data including Cost Element and Business Area. To formulate definitions of the accuracy parameters, generate DAX measures for MAE, MAPE, and RMSE (Houssein et al. 22, pp. 1-36). The use of line graphs to illustrate the actual and forecasted sales of a particular product; use of scatter plots to assess the accuracy of the forecast; and use of tables to indicate the number of errors incurred. For slicing into other finer subcategories use time and dimensional slicers. Developing plausible analytical front-end interfaces to enable time-synchronized viewing and control over the forecast accuracies to facilitate strategic business decisions.
Question 2B
Figure 2: Geographic Visualisation
(Source: Power BI)
While comparing the data for each country in each of the regions and the consolidated global data it can be deduced that the actual number of countries which made sales were less with the maximum being 62 units, 984.90 and 245.05. Thus, the total count of the country shares with respect to response rate, which is 45.59% is thus captured by 91.35 of the total count of countries. The actual counts of all the twelve data include all the continental and most of the developed countries across the world ranging from 2 upto 62. When using Power BI there is an option of geographic visualisation which can be used to show the above distribution and even possibly identify regions that score high. This would provide geographical perspective on how countries are offers and extent of dispersion, taking color intensity or size of bubble as count and sales contribution. For instance, if describing the effective date differently or sales metrics as a filter or slicer would enhance the interaction level, where the user evaluates the dispersion of sales in a particular period or for specific coefficients.
Question 3A
The synthetic dataset cleaned_Synthetic_Tourism_Data. xlsx includes the following variables: Date is to record the specific day of the following; Attraction is to list down various tourism attraction sites; Visitor_Count is to record the number of visitors on the particular day in each site; Average_Spend to record the spending of the visitors in each site; Local_Business_Revenue is to record the total revenue from tourism business in each site. Combined, these variables offer more details regarding tourism density, tourists spending and the effects on the economy for proper analysis of visitors’ profile, business income and tourism strategy and development.
Question 3B
Figure 3: Dynamic map in tourism density and its economic impact on local economies
(Source: Power BI)
Analyzing the dynamic map formulated in Power BI based on the synthetic dataset, it can be identified that the Kakadu National Park was visited by a total of 73,430 tourists and Blue Mountains having 32 tourism points had the lowest tourists’ turnout of 72,486 visitors for entire attraction type which is even 1.30% more than Blue Mountains. The visitor count for Kakadu National Park as compared to the total number of visitors for the ten attractions was a thousand and six percent. The average of the visitor counts was fluctuating in the specified range with the lowest number being 72,486 and maximum of 73,430. As a result of using the time slicer, the most popular hours for visiting are specified and the number of visits is determined, which contributes to evaluating the significant trends and effects on enterprises and helps to improve the perception of the time and money necessary for planning and investments.
Question 4A
The analysis of the magnitude of the customer base, the volume and frequency of their purchases, and the profitability of the various classifications involves different analytical tools when done with the synthetic transaction data. Firstly, according to the method called segmentation analysis it is possible to classify customers based on the factors like age, gender, and geographical location. Secondly, the RFM (Recency, Frequency, Monetary) analysis helps in defining the clients with the higher value due to their purchasing activities more easily. Last but not least, in profitability analysis, one can determine the relation between the revenues and costs which help in identification of profitable customer segments (Christy et al. 2021, pp. 1251-1257). These and other methods such as cohort analysis, Customer Lifetime Value calculation, churn prediction will help to improve knowledge and make effective decisions regarding marketing, product creation or development and CRM approaches.
Question 4B
Figure 4: Visualise Distinct Customer Segments
(Source: Power BI)
The information that was used in the above analysis is all about the financial performance of the customers and the patterns of purchases. Using the total quantity sold by the customers’ total quantity as a criterion, this research reveals that Customer 17 is the most active in this respect. But achieving the large proportionality of this customer’s contribution in terms of total quantity, 10.64% in fact, may yield great profit. Further Total Quantity reciprocally clarifies the relativity between both these variables on the generation of sales figures Total Sales. In this way, we are able to compare Total Sales and Total Quantity data and can note, for instance, that ProductID 17 can be considered an opportunity for improvement. The effort of constructing Power BI dashboard with characteristics of this knowledge will involve stacking bar oriented graphs and cards while using the measures of profitability and purchasing behavior for segmentation.
Question 5A
When you have a large and unfair data to analyze in Power BI, load the data and use the query editor. Removing duplications if data missing it should be either imputed or the data conversion should be done. Format too needs to be coherent from one format to another, such as date format and currency format. If the final database layout as a vision requires splitting or merging of rows and/or columns then it is done in an as needed basis. For example, incoherent data, data that is missing some values and big data; there should be systematic profile and validation of data. Use profiling where one can be in a position to identify the extent of the inconsistency and gain insights about the data through Power BI. Provide the right way of updating and cross-checking the data to make it healthier and ready for analysis.
Question 5B
Figure 5: Showcase Findings on a Dataset
Based on the general observation of the Power BI presentation insights described above and the other similar ones, it is apparent that the Administration department was provisioned with a total of 222 units of budget. Also, the financial breakdown of the federation budget on 1st January,2024 show that United State of America has contributed the biggest chunk with 60.81% of total budget. Thus, the following conclusions can be made based on the data considered above: the Administration department has significant impact the budget sensitisation; meanwhile, a great many of budget is used in the operations in USA. An additional resource may be the running text with the details of order presented and comments to them useful in drawing the attention of the viewer to these observations and what they imply.
References
Sharma, K., Shetty, A., Jain, A. and Dhanare, R.K., 2021, January. A Comparative Analysis on Various Business Intelligence (BI), Data Science and Data Analytics Tools. In 2021 International Conference on Computer Communication and Informatics (ICCCI) (pp. 1-11). IEEE.
Houssein, E.H., Dirar, M., Abualigah, L. and Mohamed, W.M., 2022. An efficient equilibrium optimizer with support vector regression for stock market prediction. Neural computing and applications, pp.1-36.
Christy, A.J., Umamakeswari, A., Priyatharsini, L. and Neyaa, A., 2021. RFM ranking–An effective approach to customer segmentation. Journal of King Saud University-Computer and Information Sciences, 33(10), pp.1251-1257.
Would you like to schedule a callback?
Send us a message and we will get back to you
Main Services
- Accounting Assignment Help
- Accounting Assignment Helper
- Accounting Case Study Assignment Help
- Accounting Essay Help
- Assignment Help Adelaide
- Assignment Help Auckland
- Assignment Help Ballarat
- Assignment Help Bendigo
- Assignment Help Birmingham
- Assignment Help Brisbane
- Assignment Help Bristol
- Assignment Help Calgary
- Assignment Help Canberra
- Assignment Help Darwin
- Assignment Help Dubai
- Assignment Help Dublin
- Assignment Help Geelong
- Assignment Help Gold Coast
- Assignment Help Griffith
- Assignment Help Hamilton
- Assignment Help Hobart
- Assignment Help Liverpool
- Assignment Help London
- Assignment Help Manchester
- Assignment Help New York
- Assignment Help Newcastle
- Assignment Help Oxford
- Assignment Help Perth
- Assignment Help Sydney
- Assignment Help Toowoomba
- Assignment Help Toronto
- Assignment Help Wellington
- Assignment Writing Help
- AU
- Auditing Assignment Help
- Biology Assignment Help
- Bond University Assignment Help
- Business Accounting Assignment Help
- Buy Research Paper
- CA
- Case Study Help
- Corporate Accounting Assignment Help
- Cost Accounting Assignment Help
- Coursework Writing Help
- Curtin University Assignment Help
- Deakin University Assignment Help
- Dissertation Writing Help
- Do My Accounting Assignment
- Do My Accounting Papers
- Do My Finance Assignment
- Economics Assignment Help
- Engineering Assignment Help
- Essay Writing Help
- Federation University Assignment Help
- Finance Assignment Help
- Financial Planning Assignment Help
- Flinders University Assignment Help
- Holmes Institute Assignment Help
- Humanities Assignment Help
- IT Assignment Help
- JCU Assignment Help
- Kaplan Business School Assignment Help
- La Trobe University Assignment Help
- Law Assignment Help
- Leader Institute Assignment Help
- Management Assignment Help
- Managerial Accounting Assignment Help
- Mathematics Assignment Help
- Monash University Assignment Help
- MY
- Nursing Assignment Help
- NZ
- PIA University Assignment Help
- Programming Assignment Help
- Queensland University of Technology Assignment Hel
- Research Paper Help
- Research Paper Writers
- Research Paper Writing Help
- Research Paper Writing Service
- RMIT University Assignment Help
- Science Assignment Help
- SG
- Swinburne University of Technology Assignment Help
- Thesis Writing Help
- UAE
- UK
- UNSW Assignment Help
- US
- Victoria University Assessment Help
- VIT University Assignment Help
- Write My Assignment
- Write My Research Paper
Highlights

Earn While You Learn With Us

Confidentiality Agreement

Money Back Guarantee

Live Expert Sessions

550+ Ph.D Experts

21 Step Quality Check

100% Quality

24*7 Live Help

On Time Delivery

Plagiarism-Free
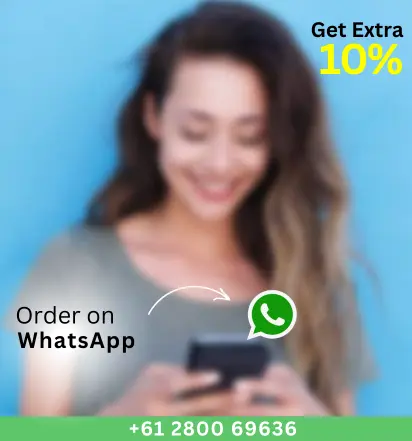
Our Samples
- MITS4001 Business Information Systems Case Study 3 Sample
- INFS5023 Information Systems for Business Case Study Sample
- PRJ5106 Research Methodology and Data Analysis Report 4 Sample
- ES5702 Planetary Health and Climate Change Report Sample
- Management Essay Sample
- MBA623 Healthcare Management PPT Sample
- GDECE103 Language and Literacy in the Early Years Report 2 Sample
- HCT343 Research Methods and Data Analysis Report Sample
- DATA4400 Data-driven Decision Making and Forecasting Report 3 Sample
- ETCH304 Diverse Literacy and Numeracy Learners Report 1 Sample
- ECX2953/ECX5953 Economics Essay Sample
- OPS911 Strategic Procurement Management Report Sample
- MBA5008 Business Research Methods Case Study 3 Sample
- TECH2200 IT Project Management Case Study 1 Sample
- ACC4001 Accounting Principles and Practices Assignment Sample
- EDU20014 Social and Emotional Learning Report Sample
- GAL613 Grief and Loss Essay Sample
- MIS608 Agile Project Management Report 2 Sample
- ICT504 IT Project Management Report 2 Sample
- TECH8000 IT Capstone Report 1 Sample
- BST714 Strategic and Operational Decision-Making Assignment Sample
- Impact of Green Supply Chain Management on The Profitability of The Retail Industry Sample
- MBA404 Consumer Behaviour and Marketing Psychology Report 3 Sample
- MBA401 People, Culture and Contemporary Leadership Report Sample
- GDECE104 Professional Teaching Practice 1 Report 2 Sample
- BUECO5903 Business Economics Assignment Part B Assignment Sample
- HI5031 Professional Issues in IS Ethics and Practice Case Study Sample
- LAW6001 Taxation Law Case Study Sample
- SITHCC001 Use Food Preparation Equipment Assignment 2 Sample
- Business Management Report Sample
- MIS605 Systems Analysis and Design Report 2 Sample
- MIS603 Microservices Architecture Report 3 Sample
- ECE6003 Contemporary Issues, Social Contexts of Learning and Social Justice Sample
- DATA4000 Introduction to Business Analytics Report 2 Sample
- HM6007 Statistics for Business Decisions/Statistics for Managers Assignment Sample
- ENGR8931 Geotechnical Engineering GE 1st Copy Sample
- HCCSSD104 Lifespan Development Report 3 Sample
- MBA402 Governance, Ethics and Sustainability Report 3 Sample
- EDU20014 Social and Emotional Learning Report Sample
- PRJ6001 Applied Project Report 1 Sample
- Information Security PG Assignment Sample
- Critically Examine and Identify the Issues within The Case Study from a Legal and Ethical Perspectiv
- Strategic and Operating Health Management Report 2 Sample
- HI6034 Enterprise Information Systems Report Sample
- ADM80001 Nanofabrication Technologies Report Sample
- MITS5004 IT Security Research Report 2 Sample
- CS4417 Software Security Report Sample
- CAS101 Community Development Report 2 Sample
- MGT602 Business Decision Analytics Report 3 Sample
- MIS500 Foundations of Information Systems Report 1 Sample
- MBA503 Operations Management and Decision-Making Models Report 3 Sample
- BUMKT5902 Marketing Mix Strategy Report Sample
- BULAW5915 Corporate Law Assignment Sample
- PSYC20042 Psychology, Wellbeing, and Resilience Website Blog Report 2 Sample
- NURBN1016 Primary Health Essay 2 Sample
- MBA401 People, Culture and Contemporary Leadership Report 3 Sample
- MBIS5009 Business Analytics Report Sample
- OMGT2327 Distribution and Freight Logistics Case Study 1 Sample
- ACCM4400 Auditing and Assurance Report Sample
- MIS608 Agile Project Management Report 2 Sample
- ACC602 Financial Accounting and Reporting Report 4 Sample
- MIS603 Microservices Architecture Case Study Sample
- MBA600 Capstone Strategy Essay 3 Sample
- NURBN3030 Management of Deteriorating Patient Report Sample
- ISYS1005 Systems Analysis and Design Report 3 Sample
- MBA505 Business Psychology Coaching and Mentoring Report Sample
- BUS5PB Principles of Business Analytics Report 1 Sample
- COIT20253 Business Intelligence using Big Data Report 1 Sample
- EASC2702 Global Climate Change Report Sample
- TCHR5003 Principles and Practices in Early Childhood Education Assignment Sample
- 6006MHR Project Management Report Sample
- PUBH1425 Life History and Determinants of Health Report Sample
- MBIS4009 Professional Practice in Information Systems Essay Sample
- HI6032 Leveraging IT for Business Advantage Report Sample
- MBA504 Introduction to Data Analytics for Business Case Study 1 Sample
- BUS6302 Integrated Marketing Communications Report 1 Sample
- Recovery Nursing Care Plan Case Study Sample
- TO5103 Global Destination Competitiveness Report Sample
- BE485 Management and Strategy Report Sample
- M20467 Strategic Management Coursework Sample
- MITS6004 Enterprise Systems Report 1 Sample
- AC400 Agribusiness Accounting Report 2 Sample
- DSMG2002 Exploring Emergency in Disaster Management Research Report 1 Sample
- ENEG11005 Introduction to Contemporary Engineering Report Sample
- TECH2300 Service and Operations Management in IT Report 3 Sample
- TECH2100 Introduction to Information Networks Report 3 Sample
- MIS605 Systems Analysis and Design Assignment 1 Sample
- PRJ5108 Project Delivery and Procurement Case Study 4 Sample
- MBA673 Business Analytics Life Cycle Report 2 Sample
- HI6008 Business Research Project Report Sample
- TECH1400 Database Design and Management Case Study Sample
- MBA501 Dynamic Strategy and Disruptive Innovation Case Study 1 Sample
- COIT20249 Professional Skills in Information Communication Technology Report 2 Sample
- BLCN29001 Construction Technology Report Sample
- EDU30059 Teaching Technologies Report 2 Sample
- ICC104 Introduction to Cloud Computing Report 3 Sample
- MBA641 Strategic Project Management Report Sample
- Social Media Audit Details Report Sample
- COIT20249 Professional Skills in Information Communication Technology Report2 Sample
- Information Security Assignment Sample
- ECUR207 Early Childhood Teacher Report Sample
- TECH8000 IT Capstone Report Sample
- MIS610 Advanced Professional Practice Report 2 Sample
- HDW204 Healthcare in the Digital World Report 1 Sample
- MGT602 Business Decision Analytics Report Sample
- MBIS4008 Business Process Management Report 2A Sample
- COU101 Theories of Counselling Essay Sample
- MBA6302 Integrated Marketing Communications Report Sample
- BUS102 Management Principles Essay 2 Sample
- BRM5002 Intercultural Awarness for Business Report Sample
- ACCY801 Accounting and Financial Management Report 2 Sample
- MIS100 Information Systems Case Study 2 Sample
- MGT502 Business Communication Report 1A Sample
- MCR006 Financial Management Assignment 3 Sample
- MBA6301 Event Management Report 3 Sample
- Principles of Economics Assignment Sample
- BE489 Analysing Organizations in the International Report Sample
- EDU10005 Indigenous Education and Perspectives Essay 2 Sample
- Rich Picture CATWOE and Root Definition Report Sample
- EDU30005 Understanding and Supporting Inclusion Report 2 Sample
- OPS909 Total Quality Management Report Sample
- MBA622 Comprehensive Healthcare Strategies Report 1 Sample
- CWB103 Interpersonal and Intercultural Negotiation Assessment 1 Sample
- MOB6110 Creating Entrepreneurial Leaders Report Sample
- MGT502 Business Communication Report 2 Sample
- ECE6012 Professional Practice Report 1 Sample
- NURBN3031 Teaching, Learning and Situational Leadership for Health Practice Sample
- HDW204 Healthcare in the Digital World Report 2 Sample
- MBA643 Project Risk, Finance, and Monitoring Report 3 Sample
- AHS205 The Australian Healthcare System within a Global Context Report 2 Sample
- GDECE102 Learning and Development Birth To Two Years Report 1 Sample
- Planning in Health and Social Care Essay Sample
- MITS4004 IT Networking and Communication Report Sample
- OPS928 Logistics Systems Assignment Sample
- Personal Improvement Plan Report Sample
- BM303 Contemporary Issues in Agribusiness Production and Management Case Study Sample
- DATA4600 Business Analytics Project Management Case Study 1 Sample
- INFS2036 Business Intelligence Report 1 Sample
- BUS101 Business Communication Essay 3 Sample
- MIS604 Requirement Engineering Report 1 Sample
- MBA6201 Quality Management Essay Sample
- MIS775 Decision Modelling for Business Analytics Report Sample
- Marketing Assignment Writing Sample
- MN7001 Summative Assessment 2 Report Sample
- Journal of Co-operative Organization and Management Sample
- EMS5RCE Risk Engineering Report 2 Sample
- PRJ5106 Research Methodology and Data Analysis Assignment 2 Sample
- MIS604 Requirements Engineering Case Study 1 Sample
- CPO442 Cybersecurity Principles and Organisational Practice Report 2 Sample
- ICT80011/40005 Energy Storage System Report Sample
- MEM603 Engineering Strategy Report 2 Sample
- BUS2008 Strategic Planning Report Sample
- NURBN2023 Pathophysiology and Pharmacology Applied to Person-Centred Nursing Essay Sample
- ENEG11005 Introduction To Contemporary Engineering Report Sample
- BUACC5931 Research and Statistical Methods for Business Assignment Sample
- EC400 Agribusiness Economics and Finance Case Study 3 Sample
- Health and Social Care in Emergencies and Disasters Report 3 Sample
- PPMP20007 Project Management Concept Report Sample
- DATA6000 Capstone: Industry Case Studies Report Sample
- OPS928 Logistics Systems Assignment Sample
- MGT610 Organisational Best Practice Case Study 2 Sample
- MITS6002 Business Analytics Research Report Sample
- CAP203 Care of the person with an acute illness Case Study 2 Sample
- HI5029 IS Project Management Report Sample
- DATA6000 Capstone Industry Case Studies Sample
- OPS802 Operations Management Report 3 Sample
- MIS609 Data Management and Analytics Case Study 1 Sample
- TECH2300 Service and Operations Management in IT Case Study 2 Sample
- TECH2200 IT Project Management Report 2 Sample
- PRJ5001 Project Management Profession Case Study 3 Sample
- MIS611 Information Systems Capstone Report 1 Sample
- ECON20039 Economics for Managers Report 2 Sample
- MGT605 Business Capstone Project Report 1 Sample
- ACCT6007 Financial Accounting Theory and Practice Report 2 Sample
- MCR007 Understanding Project Management Essay 1 Sample
- MBIS5012 Strategic Information Systems Report Sample
- MGT613 Leadership for Sustainable Futures Report Sample
- MBA633 Real-world Business Analytics and Management Report 2 Sample
- MBA600 Capstone Strategy Essay 3 Sample
- FIN311 Agricultural Accounting and Business Law Part A Report Sample
- MIS605 Systems Analysis and Design Report Sample
- DATA4000 Introduction to Business Analytics Case Study 1 Sample
- 32144 Technology Research Preparations Report 2 Sample
- TECH5300 Bitcoin Report 2 Sample
- MBA602 Small Business Administration Case Study 2 Sample
- DHI401 Digital Health and Informatics Report 2 Sample
- CSE2AIF/CSE4002 Artificial Intelligence Fundamentals Report Sample
- MBA5004 Managing Decision Making Process Report 2 Sample
- MEM601 Engineering Sustainability Report Sample
- HI5017 Managerial Accounting Report Sample
- MC7080 Digital Marketing & Social Media Report Sample
- TECH2100 Introduction to Information Networks Report 2 Sample
- MBA6103 Agile Methodology Research Report 2 Sample
- MGT502 Business Communication Report 1B Sample
- INT102 Assessment 1B Improving Communication with Vision-Impaired People Case Study Sample
- BC400 Communication and Media Management Report Sample
- EDU40002 Play and Environment Report 2 Sample
- FIT5057 Project Management Case Study 1 Sample
- STAT2009 Statistics for Managerial Decision Assignment Sample
- BIS3006 IS Capstone Industry Project B Report Sample
- MBA633 Real-world Business Analytics and Management Case Study Sample
- MGT607 Innovation, Creativity & Entrepreneurship Case Study Assignment Sample
- ACF5956 Advanced Financial Accounting Report Sample
- BUS5DWR Data Wrangling and R Report 3 Sample
- PROJ6000 Principles of Project Management Report Sample
- OPS802 Operations Management of Subway Research Report 4 Sample
- PSYC2017 Personality and Individual Differences Research Report Sample
- NURBN2022 Case Study 3 Sample
- MIS611 Information Systems Capstone 3 A Report Sample
- HS7000 Information Systems in Business Research Report Sample
- DATA4000 Introduction to Business Analytics Case Study 1 Sample
- DATA4000 Introduction to Business Analytics Report 3
- MBA504 Introduction to Data Analytics for Business Case Study 2 Sample
- TECH2400 Introduction to Cyber Security Report 2 Sample
- MIS608 Agile Project Management Report 4 Sample
- MBIS5010 Professional Practice in Information Systems Case Study 3 Sample
- BIZ102 Understanding People and Organisations Report 3 Sample
- COIT20262 Advanced Network Security Report 2 Sample
- Clinical Governance Essay 1 Sample
- MBA600 Capstone Strategy Report 2 Sample
- TCHR3001 Early Childhood Matters Report 2 Sample
- COIT20253 Business Intelligence using Big Data Report Sample
- DATA4500 Social Media Analytics Case Study Sample
- Written Assessment Report 2 Sample
- MGT501 Business Environment Report Sample
- TECH1300 Information Systems in Business Case Study 2 Sample
- MIS610 Advanced Professional Practice Report 3 Part A Sample
- CCB102 Multimedia Design Report 1 Sample
- ENEM20002 Fluid Power Engineering and Control Report Sample
- Child Development Case Study Sample
- MN691 Research Methods and Project Design Report Sample
- BIZZ201 Accounting for Decision Making Report Sample
- MBA402 Governance, Ethics and Sustainability Report 3 Sample
- PPMP20008 Initiating and Planning Projects Report 3 Part B Sample
- HA3011 Accounting Report Sample
- MITS5003 Wireless Networks and Communication Case Study Sample
- AT3 Nursing Case Study Sample
- DSMG29001 Disaster Risk Reduction Report Sample
- HEAL5004 Strategic and Operational Health Services Management Report 3 Sample
- BUS5003 Information Systems and Data Analysis Case Study 2 Sample
- PROJ-6012 Managing Information Systems, Technology Report Sample
- MBA402 Governance, Ethics and Sustainability Report 2 Sample
- BSBOPS601 Develop and Implement Business Plan Diploma Sample
- BUS2003 Data Engineering & Python Report 1 Sample
- ACCT6006 Auditing Theory and Practice Case Study Sample
- HI6032 Leveraging IT for Business Advantage Report 1 Sample
- Economics for Business Assignment Sample
- COIT20248 Information Systems Analysis & Design Report 1 Sample
- DATA4000 Introduction to Business Analytics Report 3 Sample
- MIS609 Data Management and Analytics Case Study 3 Sample
- MBA6204 Quantitative Support of Decision Making Report 2 Sample
- DSMG29002 Leadership in Emergency and Disaster Management Report 2 Sample
- PPMP20009 Control Charts and Process Mapping Assignment Sample
- OB223 Organisational Behaviour Report Sample
- MBA5008 Business Research Methods Report 2 Sample
- MIS604 Requirement Engineering Report 3 Sample
- MIS608 Agile Project Management Case Study 3 Sample
- MGT607 Innovation Creativity Entrepreneurship Case Study Sample
- BUS5VA Visual Analytics Report 3 Sample
- Journal Article Review Report 4 Sample
- MIS607 Cybersecurity Report 3 Sample
- Australian Migration Law Assignment Sample
- MLC707 Business Law Assignment Sample
- MBA404 Consumer Behaviour and Marketing Psychology Report Sample
- MBA6204 Quantitative Support of Decision Making Report 3 Sample
- INT103 Human Development Across the Lifespan Report 2 Sample
- ACC202 Contemporary Financial Accounting Report Sample
- MOB6110 Creating Entrepreneurial Leaders Report Sample
- TECH4100 UX and Design Thinking Report 2 Sample
- SBM3204 Sustainability and Ethics Case Study Sample
- BAO6504 Accounting For Management Report Sample
- PUBH6008 Capstone A Applied Research Project in Public Health Report Sample
- Principles of Supply Chain Management Report Sample
- MCR001 Economics Case Study Sample
- MBA504 Introduction to Data Analytics for Business Report 3 Sample
- ECON7200 Economic Principles Report Sample
- M33117 Public Policies and Labour Markets Report Sample
- OPS909 Total Quality Management Report 1 Sample
- HCCSSD102 Person Centred Practice Report 1 Sample
- SWOT Analysis of Chanel No5 Perfume Marketing 4ps Report
- WPDD202 Webpage Design and Development Report 4 Sample
- ICC104 Introduction to Cloud Computing Report 2 Sample
- MGT600 Management People and Teams Report 1 Sample
- ICT606 Machine Learning Report Sample
- MKTG6002 Marketing Report 3 Sample
- COIT20252 Business Process Management Report 3 Sample
- MBA6001 Investment Management Report 2 Sample
- OPS910 Linear Programming Assignment Sample
- PRJ5001 Project Management Profession Report Sample
- BULAW5916 Taxation Law and Practice Assignment Sample
- TECH2400 Introduction to Cyber Security Report 1 Sample
- DATA4700 Digital Marketing and Competitive Advantage Report 3 Sample
- PUBH6008 Capstone A Applied Research Project in Public Health Report 2 Sample
- CA7013 Sustatnability in Global Companies 2022-23 Report
- MITS5502 Developing Enterprise Systems Report Sample
- MBA404 Consumer Behaviour and Marketing Psychology Case Study 2 Sample
- BPM Final Assignment Sample
- FINM4100 Analytics in Accounting, Finance and Economics Report 2 Sample
- NURBN1012 Legal & Ethical Decision Making in Person Centred Care Sample
- MIS608 Agile Project Management Report 1 Sample
- HCCSSD103 Mental Health Case Study 3 Sample
- TUM202 Therapeutic use of Medicines Report 2 Sample
- PBHL20001 Understanding Public Health Essay 3 Sample
- MBA642 Project Initiation, Planning and Execution Report Sample
- GDECE101 Early Childhood Care and Education Report Sample
- BUS107 Business Ethics Report 3 Sample
- ENEG28001 Australian Engineering Practice Report 1 Sample
- TECH3200 Artificial Intelligence and Machine Learning in IT Report 3 Sample
- MBIS4008 Business Process Management Report Sample
- OPS928 Logistics Systems Report 2 Sample
- MANM376 International Finance Project Report Sample
- MGT601 Dynamic Leadership Report 1 Sample
- NURS2021 Dimensions of Physical and Mental Health Case Study 3 Sample
- CCS103A Counselling and Communication Skills Report Sample
- MN7002 International Business Strategy Report Sample
- HWEL2006 Social and Emotional Wellbeing Case Study 3 Sample
- Finance Mini Case Study Sample
- MGT604 Strategic Management Report 3 Sample
- WELF1014 Human Service Provision Essay Sample
- CAP203 Care of The Person With An Acute Illness Report 4 Sample
- MEE80003 Automation Strategy Case Study Sample
- HI6037 Business Analytics Fundamentals Assignment Sample
- MG304 Agriculture Value Chain Management Case Study 2 Sample
- MANM399 International Accounting and Finance Project Report Sample
- FIN600 Financial Management Case Study Sample
- PPMP20009 Case Study Sample
- Group of People Holding Papers Discussing White Laptop White Background Sample
- FE7066 Data Analysis for Global Business Coursework Sample
- TO5103 Global Destination Competitiveness Report Sample
- MIS610 Advanced Professional Practice Report 2 Sample
- NURBN2021 Nursing Essay Sample
- MBA6204 Managing the Quantitative Support of Decision Making Report 2 Sample
- MGNT803 Organisational Behaviuor and Management Report Sample
- MBA601 Fundamentals of Entrepreneurship Case Study 2 Sample
- MBA5008 Business Research Methods Report Sample
- MIS500 Foundations of Information Systems Report Sample
- MBA613 Organisational Change and Innovation Case Study 3 Sample
- DATA4900 Innovation and Creativity in Business Analytics Report 4 Sample
- Investigating The Issue of Inequality in Workplaces Essay Sample
- MECO6912 Political Public Relations Report 1 Sample
- Organisational Behaviour (OB) Essay Sample
- HI5004 Marketing Management Assignment Sample
- 7318AFE Business Data Analytics Report 2 Sample
- DATA4900 Innovation and Creativity in Business Analytics Report 3 Sample
- NUR2023 Pathophysiology, Pharmacology, and Nursing Management Case Study 2 Sample
- Local Government Area LGA Essay 2 Sample
- DATA4300 Data Security and Ethics Report 1 Sample
- TEC100 Introduction to Information Technology Report 2 Sample
- ICT101 Discrete Mathematics Report 3 Sample
- Enem28001 fea for engineering design report sample
- MBA6104 Business Process Modelling & Management Report Sample
- CAO107 Computer Architecture & Operating Systems Report 2 Sample
- IM401 Introduction to Agroinformatics Report Sample
- BE969 Research Methods in Management and Marketing Sample
- MBIS5010 Professional Practice in Information Systems Essay 2 Sample
- PLM Principles of Logistics Management Report Sample
- ADM80012 Technology Management Report 2 Sample
- DATA4400 Data-driven Decision Making and Forecasting Report 3 Sample
- EC102 Agricultural Economics Assignment Sample
- CMT218 Data Visualisation Case Study Sample
- ECUR302 Mathematics in the Early Years Report Sample
- M5011 Accounting for Management Report Sample
- TECH2200 IT Project Management Case Study Sample
- NURBN2026 Person Centered Nursing Sample
- ICT5151 Data and Information Management Report 4 Sample
- BSBPMG535 Manage Project Information and Communication Business Documents Diploma Sample
- MBA643 Project Initiation, Planning and Execution Report Sample
- INT101 Introduction to International Relations and Politics Essay Sample
- MBA404 Consumer Behaviour and Marketing Psychology Report 1 Sample
- MIS602 Data Modelling and Database Design Report 1 Sample
- MG301 Agriculture and Resource Policy Case Study Sample
- CHM108 Introduction to Business Law Report 1 Sample